-
Innovation
Generative AI tools, including text-to-3D models for object assets and text-to-image models for environment textures, enhance the realism and diversity of the simulation.
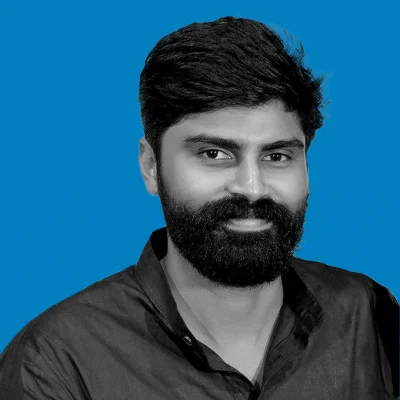
RoboCasa is a simulation framework for training generalist robots.
Robocasa
Researchers have developed a large-scale simulation framework for training general-purpose robots in diverse, everyday settings.
The framework, called RoboCasa, provides access to thousands of 3D assets over more than 150 object categories, as well as dozens of furniture and appliances that may be interacted with.
A range of generative AI tools are used to increase realism and diversity, including text-to-3D models for object assets and text-to-image models for environmental textures.
Researchers say the experiments reveal a scaling trend when large-scale imitation learning uses synthetically generated robot data, proving the potential for the use of simulation data for real-life tasks.
The details of the research done by members from the University of Texas at Austin and NVIDIA Research are available on AriXiv.
High-fidelity robot training
Recent AI breakthroughs stem from training large neural networks on massive datasets. Robotic data is scarce, unlike abundant online visual and text data for computer vision and natural language processing (NLP).
Researchers highlight that acquiring diverse, complex robotic training data remains challenging. Recent datasets have improved robot generalization in narrow domains but fall short for general-purpose robots.
Simulation offers a solution: cost-effectively generating vast synthetic data with high-fidelity simulators and automated methods like MimicGen and Optimus.
Advances in generative AI enable the creation of diverse, realistic simulations. According to researchers, effective simulation must ensure realism, diversity, and comprehensive robot datasets, yet current simulators only partially meet these criteria.
“Inspired by these advancements, we seek to develop foundation models for generally capable robots that can perform various everyday tasks. RoboCasa is designed to supply the high-quality simulation data required for training such robotics foundation models,” Yuke Zhu, lead author of the paper and a professor in the Department of Computer Science at the University, told Tech Xplore.
Advancing robotic learning
RoboCasa builds on the foundation of RoboSuite, a simulation framework introduced a few years ago. The team utilized RoboSuite’s simulation infrastructure to create RoboCasa’s detailed and immersive simulated environments.
RoboCasa offers 120 realistic scenes, dozens of appliances, and over 2,500 high-quality 3D objects across 150+ categories. It supports 100 diverse tasks and provides a substantial multi-task dataset with over 100,000 trajectories.
Generative AI tools are integral to RoboCasa. They utilize text-to-3D models for object assets, text-to-image models for environment textures, and large language models to create kitchen activities and corresponding tasks.
“We utilized generative AI tools to create diverse object assets, scenes and tasks. These AI tools significantly improved the diversity and realism of the simulated world. In addition, RoboCasa supports various robot hardware platforms and provides large datasets with over 100k trajectories for model training,” Zhu added.
Scope for improvements
The team notes that its experiments demonstrate that synthetically generated data within these simulations significantly aids in scaling robot policy learning.
RECOMMENDED ARTICLES
Despite these advancements, the team highlights certain limitations. For instance, while simulation realism has improved, transferring learned policies to real-world environments still poses challenges. Additionally, expanding the diversity and complexity of tasks to better mirror real-world variability remains a key area for improvement.
Future work will focus on enhancing the fidelity of simulations and exploring innovative methods to bridge the gap between simulated and real-world robotics applications, thereby unlocking new potential for generalist robot training and deployment.
The Blueprint Daily
Stay up-to-date on engineering, tech, space, and science news with The Blueprint.
ABOUT THE EDITOR
Jijo Malayil Jijo is an automotive and business journalist based in India. Armed with a BA in History (Honors) from St. Stephen’s College, Delhi University, and a PG diploma in Journalism from the Indian Institute of Mass Communication, Delhi, he has worked for news agencies, national newspapers, and automotive magazines. In his spare time, he likes to go off-roading, engage in political discourse, travel, and teach languages.